Identifying bird presence by analysing recordings using AI is becoming more and more widespread. It’s the audible equivalent of using a trail camera to record video of wildlife – you get to discover what’s around when you’re not. We’ve put a recorder (home-made, experimental!) in the wood, and this is about what we’ve found.
Last year I wrote a post about Merlin, a great app for your phone that helps identify birds (and pretty accurately too) as you walk around the wood. It alerted me to the presence of Spotted Flycatchers, something I wasn’t previously aware of.
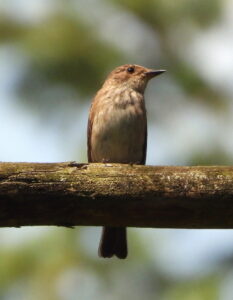
The recorder is able to record for hours or days, potentially bringing another dimension to our knowledge of the bird life in the wood.
Recording
Recording is done according to a programmed schedule, which is currently set to record for 3 minutes in every 30. This means that over a number of days (depending how long the batteries last) hundreds of recording can be made.
Analysis
BirdNET is an analysis tool that comes from the same US lab as Merlin, and it is used to identify bird calls and songs in recordings. We also use a tool with the wonderful name of Chirpity, which automatically manages the large number of recordings, feeding them to BirdNET and collating the results.
Visualisation
Chirpity creates what are called spectrograms (also known as sonograms), graphs that show the pattern of frequencies in the bird sounds. It’s these patterns that the AI tries to identify. (Strictly it’s not AI but ML ‘machine learning’ pattern matching.) The visualisation can be fascinating as it lets you “see” the bird song, and given time you can start to identify birds yourself from these patterns.
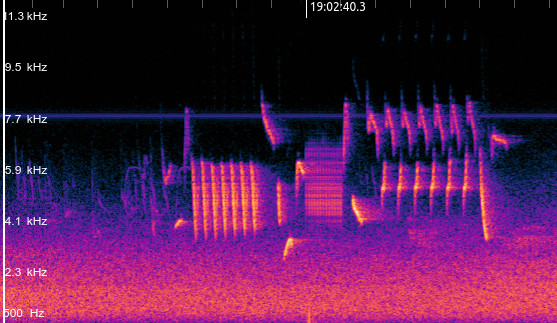
For a given species (and individuals) there is variation in the songs and calls, and the amount of that variation depends on the species. Some species can be almost immediately identifiable while others are far harder to detect a pattern. That’s the job of AI.
What can you hear?
Most birds sing below a frequency of about 12kHz, well within the normal human hearing range of between approximately 20Hz and 20kHz. “Normal” is the range we start off with as teenagers, but the upper limit drops significantly as we get older. At our recent AGM I played various frequencies to find out how the audience fared. No-one could hear above 12kHz (we had no teenagers!), most were between 8-12kHz. I turned out to have the worst upper limit at about 5kHz (not helped by tinnitus).
The spectrogram therefore gives you important information – how much of a bird’s song you can hear and particularly how much you can’t. For me, much of the Wren’s song is inaudible, but fortunately the part that is is enough to be identifiable..
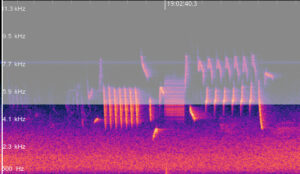
This is the actual sound that produced the spectrogram:
The song of the Goldcrest is completely beyond my range, which means I have to rely on AI to tell me they’re around.
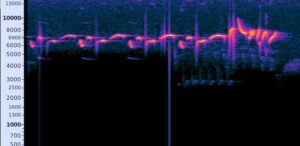
If you want to find out how high your can hear, here is an online test.
What have we detected?
Here’s a summary of what analysis has found in our first recordings, over 5 days from Feb 13th:
Species name | Max confidence | Detections |
Song Thrush | 0.991 | 89 |
Eurasian Wren | 0.985 | 10 |
Eurasian Blue Tit | 0.985 | 25 |
Great Tit | 0.974 | 11 |
European Robin | 0.973 | 88 |
Spotted Flycatcher | 0.968 | 2 |
Coal Tit | 0.956 | 9 |
Grey Partridge | 0.948 | 1 |
Yellowhammer | 0.938 | 5 |
Redwing | 0.93 | 7 |
Eurasian Siskin | 0.919 | 1 |
Eurasian Blackbird | 0.888 | 2 |
Hawfinch | 0.875 | 1 |
Eurasian Green Woodpecker | 0.874 | 2 |
Carrion Crow | 0.864 | 4 |
Long-tailed Tit | 0.847 | 1 |
Dunnock | 0.76 | 3 |
Great Spotted Woodpecker | 0.75 | 3 |
Water Rail | 0.7 | 1 |
Marsh Tit | 0.661 | 1 |
Crested Tit | 0.601 | 1 |
Common Woodpigeon | 0.572 | 1 |
Eurasian/Green-winged Teal | 0.556 | 1 |
Eurasian Bullfinch | 0.527 | 1 |
Common Raven | 0.525 | 1 |
Rook | 0.513 | 1 |
Mallard | 0.502 | 1 |
Clearly there are some questionable entries here. AI detection is by no means foolproof, so how do we decide what to believe?
High confidence together with multiple detections makes identification pretty certain, like the Robin and Song Thrush. Also, prior knowledge of species known to be present helps with lower confidence figures, such as Blackbird and Dunnock. Everything that’s left is not impossible (although not necessarily likely, like the Crested Tit or Water Rail).
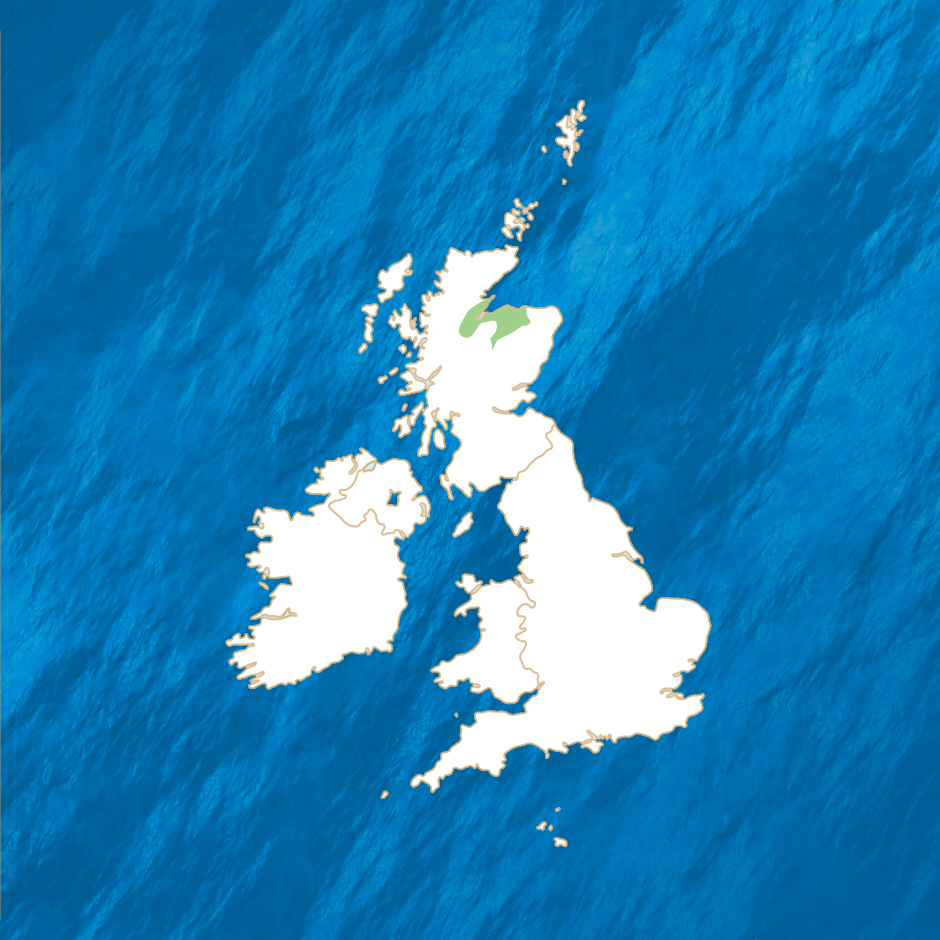
To help with further verification, we can listen to the actual recording to see if we can do better than the AI – which of course needs familiarity with the song, and the ability to hear it.
Ultimately when all else fails, we should just treat the unexpected as an invitation to investigate further, with perhaps good old-fashioned technology – binoculars and camera.
Our recorder will be moved around the wood as time goes on. Preliminary results show variations in different parts, and different species will appear at different times of the year. Keep tuned for further bulletins!
If you find this interesting, post a comment to let me know.